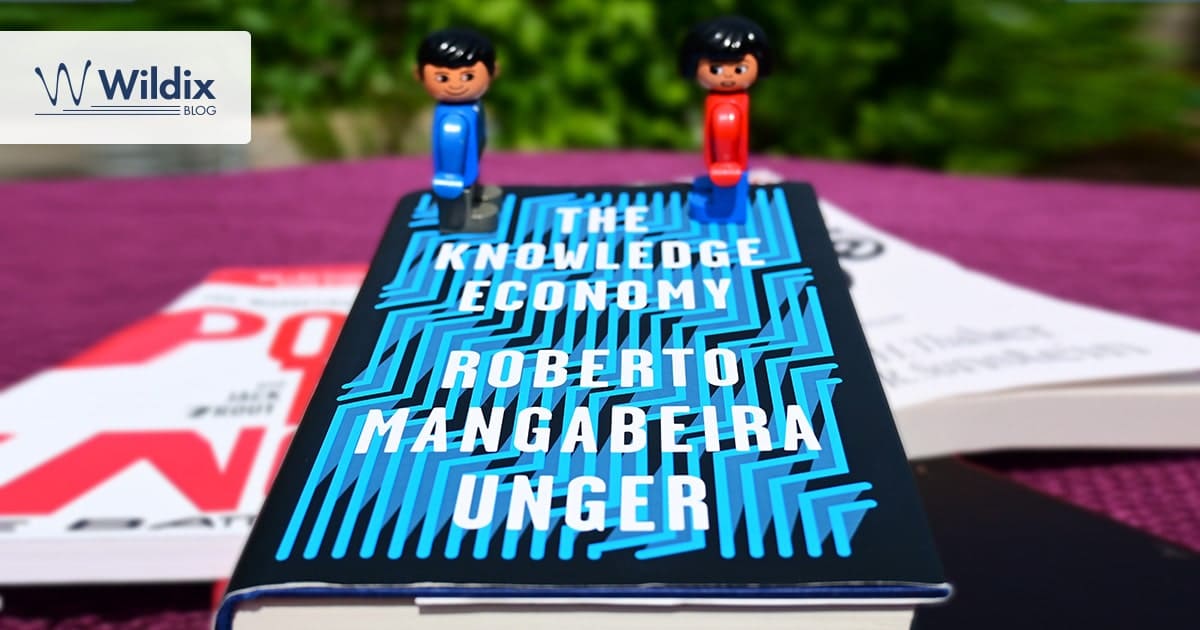
The knowledge economy is a concept where the knowledge contained within a company or even an individual drives it, rather than its actual physical output. This is contrasted with an agrarian society (agricultural/food) and a manufacturing economy (physical products), and it differs from a service economy in that knowledge-intensive products become the output, not a physical service. The knowledge economy is often considered a major component of a modern developed economy.
Typically, knowledge economies have highly skilled and specialised workforces and large institutions (whether enterprises or centres of learning) that capitalise on this knowledge.
But the rise of AI threatens all of that.
The Current Knowledge Economy
At the moment, knowledge is generally held by humans. You pay someone to write an article, for example, or create new ideas for marketing campaigns. Your sales teams are human, sending emails, responding to clients, and delivering products.
Your setup teams are human. Your development teams are all human. Accounting, payroll, HR — all human.
That’s because knowledge is siloed within those people. You pay them for the value they bring to your organization, and they use technology to enhance their value. They assess recordings, check data and make decisions. And they store those results in large databases, and companies use that knowledge.
And that’s where the value in people lies: They make decisions based on the knowledge they have.
Despite major advances in how accessible knowledge has become, we’re still limited by the ways humans can absorb and reuse that information. Other limitations include being able to reject false information (with varying degrees of success), understanding prioritisation and being able to make random choices when necessary.
On completion of a Ph.D., students are often told that they are now the foremost experts in that particular field of expertise, whether it’s about the differences between ancient and modern viewers of Greek urns or the use of lanthanide-based crown thioethers in phase boundary transitions (basically moving molecules between petrol and water).
Yet their knowledge doesn’t automatically translate into being paid more. So there’s another component: How valuable is their knowledge to a company?
A chemist’s knowledge may be incredibly lucrative because their research could lead to new ways of creating drugs, catalysts or pesticides, for example. Someone researching classics may not have a similar level of commercial value directly from their Ph.D. Similarly, someone who has spent 20 years in marketing may have more commercially relevant knowledge than someone who has only a year under their belt.
Then there’s the ease of replacement: How quickly can you replace a person with that knowledge? Someone with rare, specialised knowledge may be worth more than someone with a broader skillset because replacing them is harder and therefore costs more.
These are all big generalisations, of course.
What this means, however, is that we have the rarity of specialisation, difficulty of replacement and financial appeal as the three main guiding principles as to how much a person is “worth” to a company in the knowledge economy.
The Current Limitations of AI
One of the main reasons computers struggle to recreate human thought patterns is because humans analyse information heuristically.
Ask a computer what a box is, and it would find it difficult to establish parameters that accurately defined what a box is.
In short, humans don’t need to know every single possible version of a box to understand that something is a box. We make note of key aspects of it and apply that information to our lives. This can lead to interesting philosophical debates as to what point does a box become a tray, for example. Can a box be both a box and a tray?
We’d also be able to reject items that kind of look like boxes but clearly aren’t boxes. A book might be an example.
This ability to reject false information heavily separates humans from AI at the moment.
One possible solution is to use the internet as a huge dataset. But if an AI is introduced to the internet, it often becomes the worst representation of itself. That’s because vitriol on the internet is easy to find, and the most vitriolic users are often the most frequent contributors. If an AI weights content according to the amount of similar content it finds, it can produce highly bigoted information itself. This has become a problem in several fields, including recruiting.
Famously, Amazon stopped its AI recruitment tool once it realised that the original data it used in its AI/machine learning model was flawed from the outset. It had identified male dominance as being preferred (because of how Amazon had recruited in the past), and it downgraded certain phrases (such as “women’s” and the name of two all-female schools) even though it had been told not to focus on gender.
This means information streams must be partitioned out to train an AI. Deficiencies in the model ensure the AI will produce results that aren’t intended — much like the issues with training humans.
Garbage in = garbage out
The Many Failures of AI
Even in the military, where split-second decision-making is required, we’re hesitant to use automation to make decisions even faster. Reports of humans being removed from the decision-making process pushed the UN to propose a ban on AI-led decision-making in battle. While that failed due to pressure from the UK, the US and Russia, there is a possibility that the next incident will push nations further away from military AI.
Vehicles using AI driverless technologies have long claimed they are safer than modern cars. But they can be defeated by a ring of salt around the car. AI hasn’t been all it’s cracked up to be.
With ChatGPT, for example, it’s still possible to spot AI-written essays due to the way the models work — they rely on stock phrasing, and their knowledge of events post-2021 is limited. Search engines are already looking at ways to identify and eliminate AI-written content from the web, reasoning that most people will be looking for human-written content and ideas.
At the moment, AI can only regurgitate existing ideas that it finds. Consequently, it can’t create new content, such as quotes and new theories and ideas.
AI that works more autonomously, such as AutoGPT, can handle less structured questions to deliver results. However, it’s not that clear where it gets its information from sometimes or how it arrives at its conclusions. This can create problems for those who require accountability in their fields. After all, if an AI model recommends you buy a stock and that stock crashes, who is accountable for that decision? Or makes a healthcare decision and it’s wrong?
What Would the Destruction of the Knowledge Economy Look Like?
There is the danger that, as AI becomes more embedded, there will be a focus not on what people know but what AI tools can they use.
This would start to destroy the knowledge economy buildup by gradually moving highly complex knowledge bases to algorithms. Instead of hiring a developer, you’d hire run-time on an AI program to deliver code that’s accurate and fit for purpose. Instead of hiring a content writer, you’ll insert your requirements into a knowledge base, and it’ll create a topic for you.
The use of AI makes access to knowledge a level playing field, in theory.
What actually may happen is that knowledge becomes even more distilled into big business, and the cost of buying into that big business and challenging it will become progressively more difficult. New AI providers will be assessed by other AIs, and if their knowledge is deemed useful, they’ll be acquired quickly. If it’s not, they’ll be subject to intense scrutiny and gradually wither.
The use of AI may gradually erode the ability of humans to express creativity and make gut instincts. We already have software that claims to make judgments of how good an advert or a piece of content is based on eye movements and preferred placement. We have algorithms that serve up content based on user preferences (“you might also like”). Some algorithms can help you plan your meals or manage your schedule.
And eventually, maybe AI will start making actual judgments about which employees are good to retain. Managers will become redundant, and employee feedback may be automated. Eventually, AI could conceivably run every aspect of a company, from automated vehicles and delivery services to checkouts and security.
Is It Hopeless?
Perhaps we’re painting too bleak a picture.
AI certainly has made an impact, but do we have enough processing power to handle trillions upon trillions of interactions around the world for every business?
Probably not.
At the moment, we’re producing computer chips (AMD, Intel) under the 3 nanometer node. This doesn’t actually bear much resemblance to any individual transistor size but is a naming convention. There are plans for a 2 nanometer node, and beyond that, a 1.4 nanometer node. Beyond that, it’s not completely clear how they’ll be able to continue the process.
There’s only so small we can go with our current technology before we start running into huge problems with quantum effects (quantum tunneling, to be precise). These effects are already a problem, and they’ll worsen as the technology shrinks.
So, the only way to start relying more on AI is to gradually increase the number of processors available to it. But that takes huge amounts of energy, and there’s already an energy crisis as oil stocks run out and there’s a marked lack of fuel available to power everything on the grid already.
So we can’t really justify the increase in energy consumption to sell more products right now. And we can’t really justify the additional costs to the cost of living. And we can’t justify the cost of the AI tools that may eventually force people out of their jobs.
Consequently, more advanced AI is priced out of the market. So knowledge-driven jobs are probably safe for now.
Maybe …
For more insight on cybersecurity and tech, subscribe to receive our magazine for free!